The Mixtral language model is a Large Language Model (LLM) contributing to groundbreaking strides in artificial intelligence. Rooted in deep learning algorithms and trained on expansive textual datasets, these models can discern context, grasp nuance, and produce startlingly coherent and relevant responses. The impact of LLMs extends far beyond mere text generation; they are revolutionizing how humans interact with machines. By providing more natural and intuitive user experiences, LLMs are narrowing the chasm between human cognition and machine processing, enabling machines to assist with diverse tasks, including customer service and content creation. Through this ongoing evolution, LLMs are not just reshaping our interactions with technology, they are redefining our digital existence.
Understanding Large Language Models
Large Language Models (LLMs), such as Mixtral, are advanced artificial intelligence programs capable of processing, understanding, and generating human language that is coherently and contextually relevant. These complex algorithms are essentially vast neural networks trained on expansive text datasets, learning linguistic patterns, structures, and the intricacies of language nuances.
Training an LLM involves feeding it with large amounts of text and adjusting the model’s parameters through iterative processes until it can predict or generate the next word in a sequence with high accuracy. It allows LLMs not only to complete sentences or paragraphs but also to create entire articles, engage in detailed conversations, and even write poetry, emulating the subtleties of human communication.
The Interaction Enhancement
Through the use of LLMs, the quality of human-machine communication has seen significant improvements. Machines equipped with these models can engage users in fluid, natural-sounding dialogues, closely mimicking human conversation. This heightened interaction is especially advantageous in customer service applications where bots can accurately understand and respond to customer inquiries, reducing wait times and improving customer satisfaction.
Virtual assistants, another prominent use case, are now more adept at understanding context and user intent. It allows them to provide personalized support, perform complex tasks, and anticipate user needs. These advancements underline the potential of LLMs to streamline mundane tasks and provide a level of interaction that approaches genuine human empathic connection.
The Human Aspect
The fear of automation replacing human labor is prevalent, and LLMs present a narrative that is more about aid than displacement. Rather than supplanting human workers, LLMs augment human capabilities, allowing individuals to focus on more creative and strategic tasks. For instance, they can help legal professionals by swiftly sifting through and summarizing vast legal documents, enabling lawyers to direct their efforts toward critical thinking and client interactions.
In educational settings, LLMs can provide supplementary tutoring, offering personalized learning experiences while teachers devote more time to individualized student mentorship. The integration of LLMs into the workforce is thus reshaping the labor landscape, propelling society towards a future where human creativity and machine efficiency coalesce to increase productivity and foster job satisfaction.
Demystifying LLMs
LLMs often evoke misconceptions and provoke fears regarding the potential for job displacement, overreliance on automation, and loss of privacy. As much as LLMs have revolutionized communication, they are tools needing conscious and ethical application. Ethical development and deployment of LLMs demand stringent guidelines to prevent biases in AI responses, ensure data privacy, and avoid misinformation.
Although LLMs are astonishing in their capabilities, they are not infallible; they can generate incorrect or nonsensical information, lack common-sense reasoning, and are prone to reflecting biases in their training data. Addressing these issues through transparency, cross-disciplinary cooperation, and ongoing research is crucial in harnessing LLMs’ potential while safeguarding against their shortcomings and ensuring they complement human intellect rather than attempt to replicate it.
Conclusion
LLMs like Mixtral are shaping a future where the line between human and machine intelligence becomes increasingly blurred. While they pose challenges and stir ethical discussions, their potential to enhance human-machine interactions is undeniable. As tools that can boost efficiency and creativity, LLMs hold great promise for various industries, yet they also require careful consideration and responsible use to maximize benefits and minimize risks. By demystifying LLMs and emphasizing their role as augmentative rather than replaceable, we can embrace a symbiotic relationship with technology, where human ingenuity is amplified through AI collaboration.
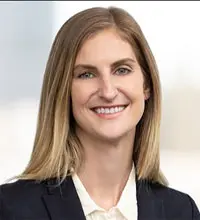
Caroline is a dedicated writer with a passion for keeping readers informed. Specializing in providing the latest news updates and unbiased reviews, she strives to deliver accurate and insightful content. With a keen eye for detail and a commitment to journalistic integrity, Caroline ensures that her readers are always well-informed. Stay tuned for her latest articles to stay up-to-date on current events and trends.